Link: https://rajivsethi.substack.com/p/the-new-mortgage-fee-structure
Graphic:
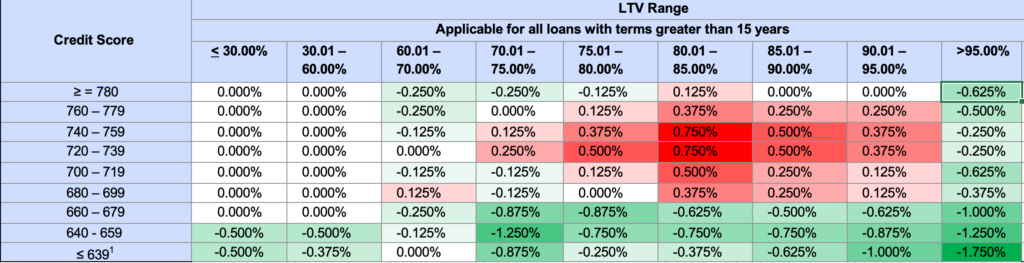
Excerpt:
Notice that credit scores below 639 have been consolidated into a single row, and those above 740 have been split into three. In addition, loan-to-value ratios at 60% and below are now in two separate columns rather than one. This makes direct visual comparisons a little difficult, but one thing is clear—at no level of the loan-to-value ratio does someone with a lower credit score pay less than someone with a higher score. That is, one cannot gain by sabotaging one’s own credit rating.
In general, there are two types of borrowers who stand to gain under the new fee structure: those with low credit scores, and those with low down payments. In fact, those at the top of the credit score distribution gain under the new structure if they have down payments less than 5% of the value of the home. The following table shows gains and losses relative to the old structure, with reduced fees in green and elevated ones in red (a comparable color-coded chart with somewhat different cells may be found here):
What might the rationale be for rewarding those making especially low down payments? Perhaps the goal is to make housing more affordable for people without substantial accumulated savings or inherited wealth. But there will be an unintended consequence as those with reasonably high credit scores and substantial wealth choose to lower their down payments strategically in order to benefit from lower fees. They may do so by simply borrowing more for any given property, or buying more expensive properties relative to their accumulated savings. The incentive to do so will be strongest for those hit hardest by the changes, with credit scores in the 720-760 range and down payments between 15% and 20%.
Author(s): Rajiv Sethi
Publication Date: 23 Apr 2023
Publication Site: Imperfect Information at Substack