Link: https://tsangchungshu.medium.com/statistics-with-zhuangzi-b75910c72e50
Graphic:
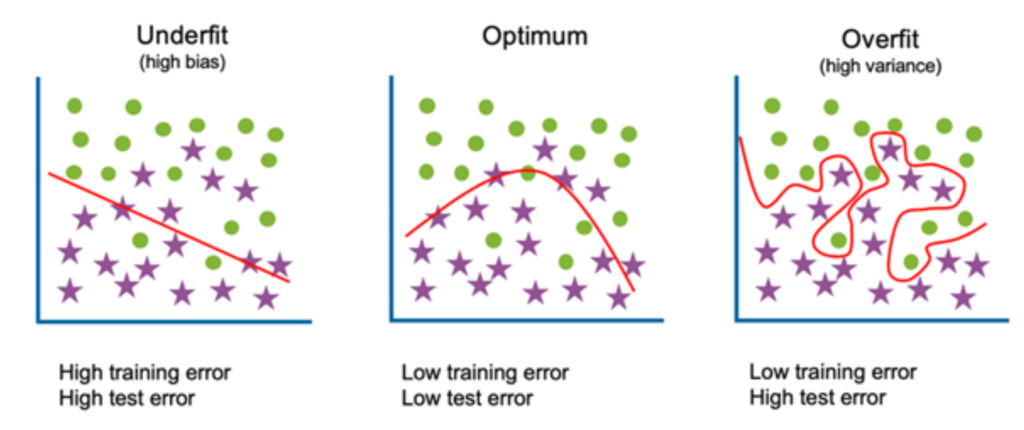
Excerpt:
The next section is another gratuitous dunk on Confucius, but it’s also a warning about the perils of seeing strict linear relationships where there are none. Not only will you continually be disappointed/frustrated, you won’t know why.
In this story, Laozi suggests that Confucius’ model of a world in which every additional unit of virtue accumulated will receive its corresponding unit of social recognition is clearly not applicable to the age in which they lived.
Moreover, this results in a temptation is to blame others for not living up to your model. Thus, in the years following the 2007 crash, Lehman brothers were apostrophised for their greed, but in reality all they had done was respond as best they could to the incentives that society gave them. If we wanted them to behave less irresponsibly, we should have pushed government to adjust their incentives. They did precisely what we paid them to. If we didn’t want this outcome, we should have anticipated it and paid for something else.
Author(s): Ts’ang Chung-shu
Publication Date: 20 Sept 2021
Publication Site: Medium