Link: https://nightingaledvs.com/data-visualization-using-chatgpt-to-code/
Graphic:
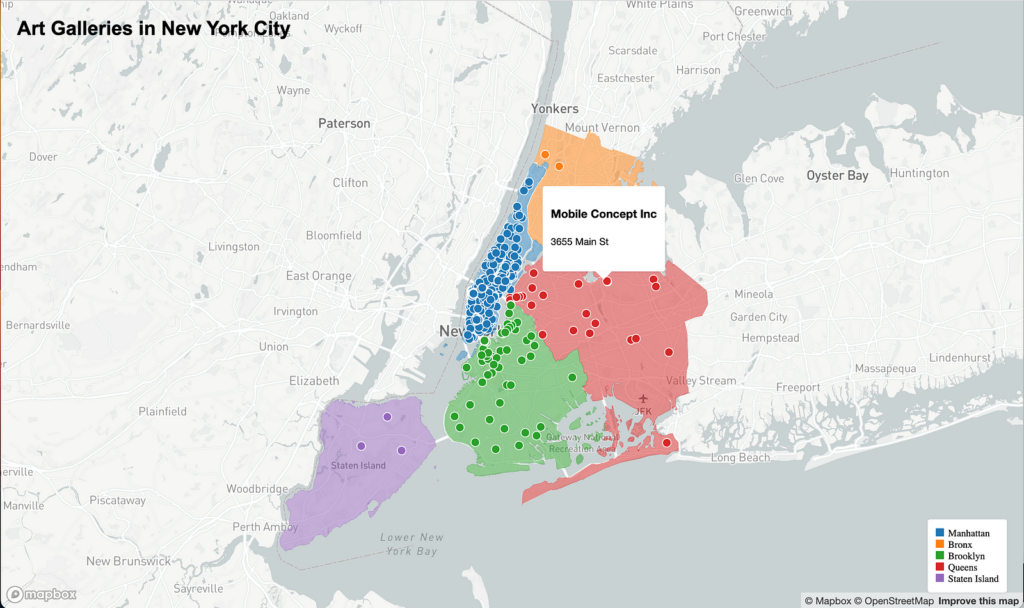
Excerpt:
Data visualization is cool but at the same time it’s bit daunting that I need to know lots of tech stacks to actually implement it.
I totally agree that even when I was studying data visualization, I spent a pretty substantial amount of time learning how to code, handle web hosting, work with Python, SQL, and more, all while absorbing knowledge on information visualization.
Thankfully, we no longer need to deep dive into technical gatekeepers in this field. This doesn’t mean that technical knowledge is not valuable, but rather that we no longer need to be intimidated by technology because AI can spoon-feed us knowledge and do the heavy lifting for us. Are you excited? Let’s get started!
I’m going to build the data visualization that one of my students posted on weekly write-up homework.
Author(s): Soonk Paik
Publication Date: 4 April 2023
Publication Site: Nightingale